A Research Voyage for Psychologists
Guillermo Campitelli
From traditional methods to model comparison and Bayesian inference
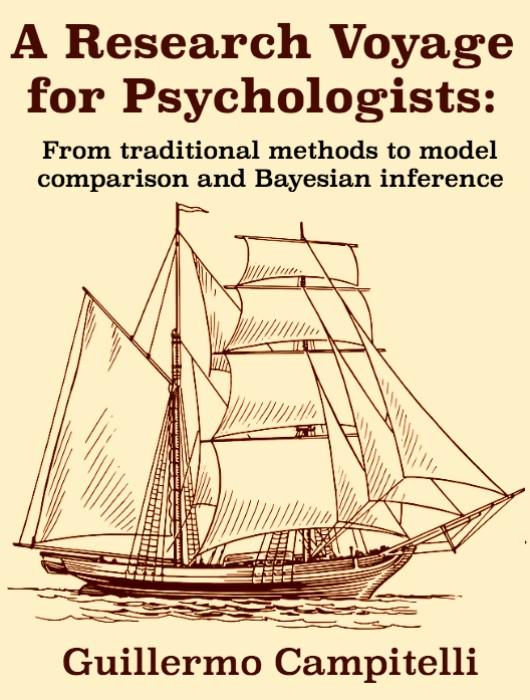
Top Hat with Interactive eText
requires a join code from instructor
$40
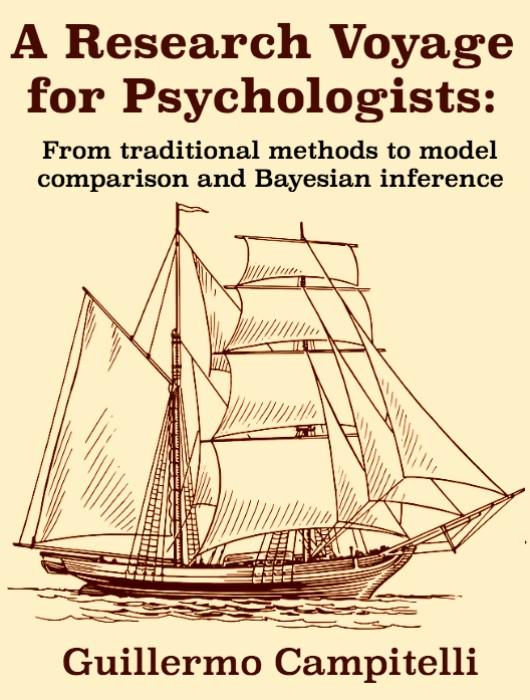
Table of Contents for A Research Voyage for Psychologists
- A Research Voyage for Psychologists