Statistics Without Tears for Business Management
Stanley Buchin
This text presents a different approach to statistical analysis that avoids the headaches of math-intensive techniques in favor of graphics and computer programs.
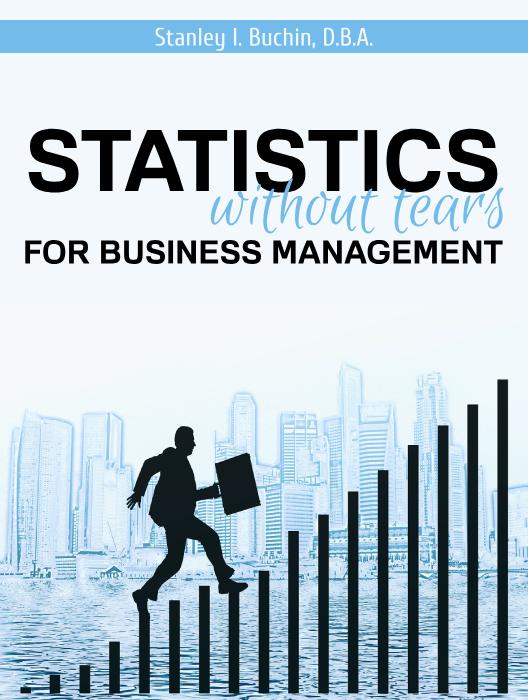
Top Hat with Interactive eText
requires a join code from instructor
$50
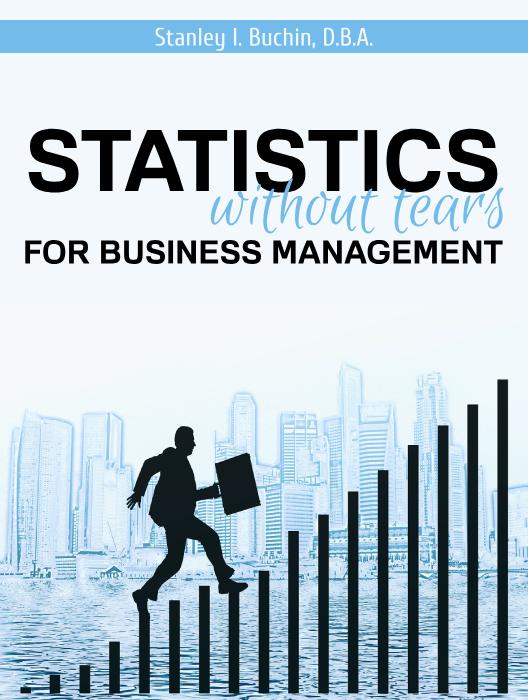
Table of Contents for Statistics Without Tears for Business Management
- Statistics Without Tears for Business Management